Artificial Intelligence in Healthcare: Biotech or Tech-bio
The increasingly broad adoption of artificial intelligence technologies is having a wide-ranging impact in the healthcare market. This is already observed across health tech projects, and especially in the field of radiology, with hundreds of algorithms approved for commercial use in the US. Under the term tech-bio (as opposed to biotech), new AI focused companies are attempting to transform the drug development and health services markets. A gradual convergence of biotech and tech-bio is in process and will continue for the years to come. We believe that it will generate numerous attractive investment opportunities, but investors targeting this opportunity should understand where we are in the tech development cycle, what business models will likely triumph and what the current limitations are in the use of AI for healthcare.
Recent developments in the use of artificial intelligence solutions applied to the health sector are transforming almost every field of the industry, from the identification, design, and development of new therapeutics to diagnostics or the provision and management of almost every service and product in the healthcare market.
AI applied to drug discovery.
Computational methods have been applied to drug development for some time:
- In the early 1980s Computer Aided Drug Design (“CADD1”) was considered a revolution as it enabled the creation of virtual models of drug molecules and their targets2 to see how well they might bind together.
- In the 1990s Quantitative Structure Activity Relationship (“QSAR3 methods were adopted to reveal relationships between biological activities and the structural properties of chemical compounds.
- Developments accelerated in the 2010s when the wider adoption of Deep Learning Techniques demonstrated real benefit as in the QSAR challenge set by the pharma-giant Merck in 20134 or in the Critical Assessment for Structure Prediction competition in 20185 when Deepmind´s AlphaFold team, using deep neural networks, was able to predict the shape of twenty-five out of the forty-three most difficult proteins based on their DNA, by training the model against a set of known proteins.
- In another remarkable development, AlphaFold2 was opened for public use in 2022 triggering an acceleration of advanced AI tools deployed in fundamental and applied biological research.
As a result of the broader adoption of AI tools in most aspects of the drug development process according to the FDA (the US pharmaceutical and health regulator), in 2021 more than 100 new drug and biological submissions reported using components of AI/machine-learning.6
According to Alacrita3 research, the contribution of AI to drug discovery could be promising in several ways:
- Target identification: the identification of molecules (like human proteins or genes) or pathways in the human body that are involved in a disease, which can be modulated by drugs to achieve a therapeutic benefit, requires managing large amounts of biomedical data from fundamental research, disease mechanism and patient-derived data.
- Protein structure prediction: three dimensional structures of the protein are essential to understanding how a promising potential drug binds to the target protein. Despite the progress achieved by Alphafold2, protein ligand7 prediction remains very challenging due to its complexity.
- Virtual screening: the recent growth of chemical libraries containing billions of molecules or chemical particles (potential new drugs) represents an opportunity that requires efficient and effective virtual screening methods. Conventional techniques may take a long time to identify a “lead”8 with suitable properties for clinical progression.
- Chemical and functional properties: it is essential to understand how the body absorbs, distributes, metabolizes, and eliminates a drug. As indicated earlier, notable progress has been achieved using deep neural networks applied to QSAR, however, further efforts remain necessary to predict abnormal results across various protein families.
- De novo drug design: as opposed to screening against a library of preexisting compounds, AI assisted de novo design is a more complex process that can generate a wide variety of new structures. As of September of 2023, only one entirely AI designed drug by Insilico Medicine has successful entered Phase 2 trials.
- Drug repurposing: consists of using the wealth of data surrounding an approved or trialed drug to repurpose the drugs into new directions.
Against this promising background, recent setbacks in some AI centric companies’ clinical trials, like those of BenevolentAI or Exscentia, show that the progress made in ligand design and synthesis is not enough and that we need to further advance our understanding of biology and in the generation of data related to efficacy and safety points9.
AI applied to the pharma sector.
McKinsey and Co10 recently published a report evaluating how pharma companies could extract value from the adoption of generative AI solutions in various aspects of their businesses, estimating that the technology could generate between $60B-$110B a year across the following areas of activity as shown in chart 1:
- Research and early discovery: helping identify targets singling out promising leads and assisting in preclinical testing to determine effectiveness. Overall, McKinsey estimates about a 10% increase in the probability of success for trials and a 20% reduction in cost and duration.
- Clinical trials: streamlining clinical trial processes and generating up to 50% cost reductions, by reducing the time it takes to conduct a trial by more than 12 months and increasing the projects net present value by at least by 20% through enhanced health authority interactions, quality control and improved signal management.
- Operational performance: improving all aspects of the operations value chain, sourcing, manufacturing, quality control and supply chain.
- Commercial performance: improving various aspects of the interaction with care providers, pharmacists, insurers, and patients.
- Medical affairs: by supporting patient care by enabling trusted scientific exchanges between pharma companies and healthcare providers that generate strategic insights for the client.
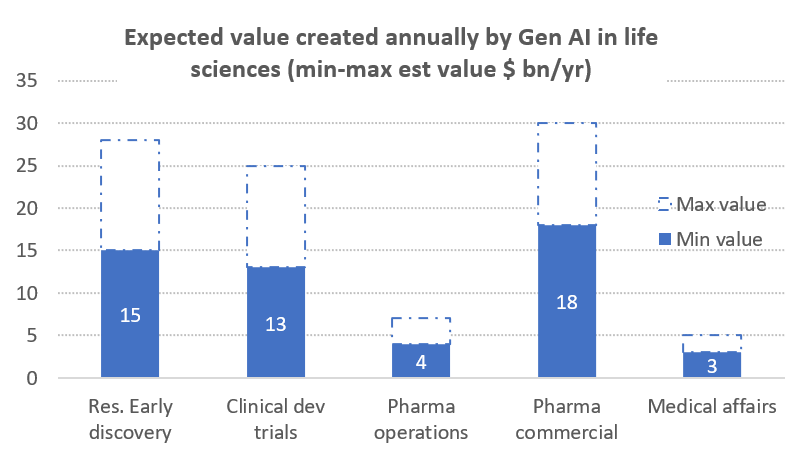
Source: McKinsey and Co
AI in healthcare for investors.
The past year was a challenging year for technology investors. In Q4 2023, biopharma venture funding dipped to $6.3 billion, a decrease from $7.8 billion in Q3 and a drop from $36.7 billion in 2022 to $29.9 billion in 2023, indicating a cautious VC climate11. The AI space is arguably one of the few where despite the venture capital downturn, we have seen activity across a broader spectrum of areas. Funding levels and number of deals have been lower in 2023 than in prior years, yet investors continue to be interested in various applications of AI technologies across multiple healthcare markets as shown in chart 2:
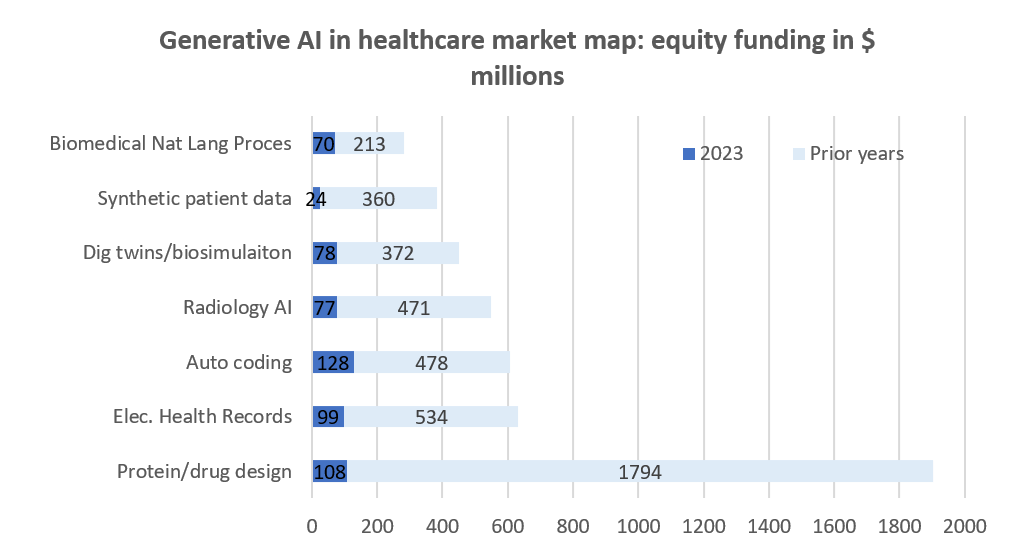
Source: CB insights
Nevertheless, the adoption of AI continues its expansion and is particularly successful in the field of enabled medical devices in the US. In December of 2013 Healthimaging12 reported that the FDA had already approved 700 AI healthcare algorithms, more than 76% of which are in the field of radiology with 521 cleared to date followed by cardiology (71), neurology (16), hematology (14) and gastro-urology (10).
For all the reasons indicated above, AI has the potential to yield substantial and positive impact in the healthcare industry. As investors, we are excited to be already benefitting from opportunities in this field. At the same time, when thinking about making new investments in AI focused companies we believe one should bear in mind some considerations:
- A multiplicity of new AI software life sciences focused companies is being launched and funded. Some are closer to a software company than to a biotech start up (more tech-bio than biotech). However, their aim is to transform the biotech or healthcare market. To achieve this ambitious goal, they will need to incorporate the knowledge, experience and skills required to navigate the complex life sciences ecosystem. A deeper convergence of tech-bio and biotech is likely to occur in the years to come.
- In the field of therapeutics, there are still significant limitations in the application of AI tools across all aspects of the drug development process. Thus, it is critical to understand these limitations and how new AI tools can effectively enhance the capacity of a biotech team to accelerate new products to market.
- Despite all this progress, bringing a new drug to market remains a capital and time intensive effort that requires a syndicated exercise among investors. Very ambitious and transformational projects will still need large support from a variety of market participants.
- AI tools and libraries are being developed at a fast pace, and at times with limited barriers to entry. Investors should understand who the current players are that, through their federated business models or access to proprietary data bases, will not only successfully develop a product but also be able to retain a competitive advantage over time.
- AI solutions are already present in the market and offer great value, notably in the areas of radiology and pathology. Beyond the medical and societal value of these solutions, it is important to understand and assess how to capture this value through targeting the right technologies and assessing the stage of maturity of the company.
It is an exciting time of progress and opportunity in healthcare. We are very optimistic about how tech-bio can propel biotech, but it is also important to understand its limitations and correctly identify winning strategies from an investor perspective.
1 “Designing drugs with computers” Marcia Bartusak, Discover, August 1981
2 The protein or molecule in the body that is involved in a disease process and that a drug can interact with
3 Kwon, S., Bae, H., Jo, J. et al. Comprehensive ensemble in QSAR prediction for drug discovery. BMC Bioinformatics 20, 521 (2019) https://doi.org/10.1186/s12859-019-3135-4
4 “AI in small molecule drug discovery: black box or crystal ball” Alacrita 2023
5 “The Coming Wave” Mustafa Suleyman 2023
6 https://www.fda.gov/science-research/science-and-research-special-topics/artificial-intelligence-and-machine-learning-aiml-drug-development
7 Molecules that bind specifically to a protein to carry out certain functions, such as activating, inhibiting, or modifying its activity. Protein ligands can be drugs designed to interact with a specific human protein (target).
8 Chemical compound that shows promise in early testing for becoming a new drug. The lead has desirable properties that affect the target in a way that could potentially treat a disease, but it will need more modification and testing to ensure it’s effective, safe, and can be developed into a drug suitable for patients.
9 “Artificial intelligence in drug discovery: what is realistic, what are illusions? Part 1: Ways to make an impact, and why we are not there yet.”
10 “Generative AI in the pharmaceutical industry: moving from hype to reality” McKinsey and co; January 2024
11 “Biopharma report: Q4 2023” Pitchbook
12 https://healthimaging.com/topics/artificial-intelligence/fda-has-now-cleared-700-ai-healthcare-algorithms-more-76-radiology
IMPORTANT NOTICE:
This document has been prepared by Altamar CAM Partners S.L. (together with its affiliates “AltamarCAM“) for information and illustrative purposes only, as a general market commentary and it is intended for the exclusive use by its recipient. If you have not received this document from AltamarCAM you should not read, use, copy or disclose it.
The information contained herein reflects, as of the date hereof, the views of AltamarCAM, which may change at any time without notice and with no obligation to update or to ensure that any updates are brought to your attention.
This document is based on sources believed to be reliable and has been prepared with utmost care to avoid it being unclear, ambiguous or misleading. However, no representation or warranty is made as of its truthfulness, accuracy or completeness and you should not rely on it as if it were. AltamarCAM does not accept any responsibility for the information contained in this document.
This document may contain projections, expectations, estimates, opinions or subjective judgments that must be interpreted as such and never as a representation or warranty of results, returns or profits, present or future. To the extent that this document contains statements about future performance such statements are forward looking and subject to a number of risks and uncertainties.
This document is a general market commentary only, and should not be construed as any form of regulated advice, investment offer, solicitation or recommendation. Alternative investments can be highly illiquid, are speculative and may not be suitable for all investors. Investing in alternative investments is only intended for experienced and sophisticated investors who are willing to bear the high economic risks associated with such an investment. Prospective investors of any alternative investment should refer to the specific fund prospectus and regulations which will describe the specific risks and considerations associated with a specific alternative investment. Investors should carefully review and consider potential risks before investing. No person or entity who receives this document should take an investment decision without receiving previous legal, tax and financial advice on a particularized basis.
Neither AltamarCAM nor its group companies, or their respective shareholders, directors, managers, employees or advisors, assume any responsibility for the integrity and accuracy of the information contained herein, nor for the decisions that the addressees of this document may adopt based on this document or the information contained herein.
This document is strictly confidential and must not be reproduced, or in any other way disclosed, in whole or in part, without the prior written consent of AltamarCAM.